Raul Fraile: How GZIP compression works
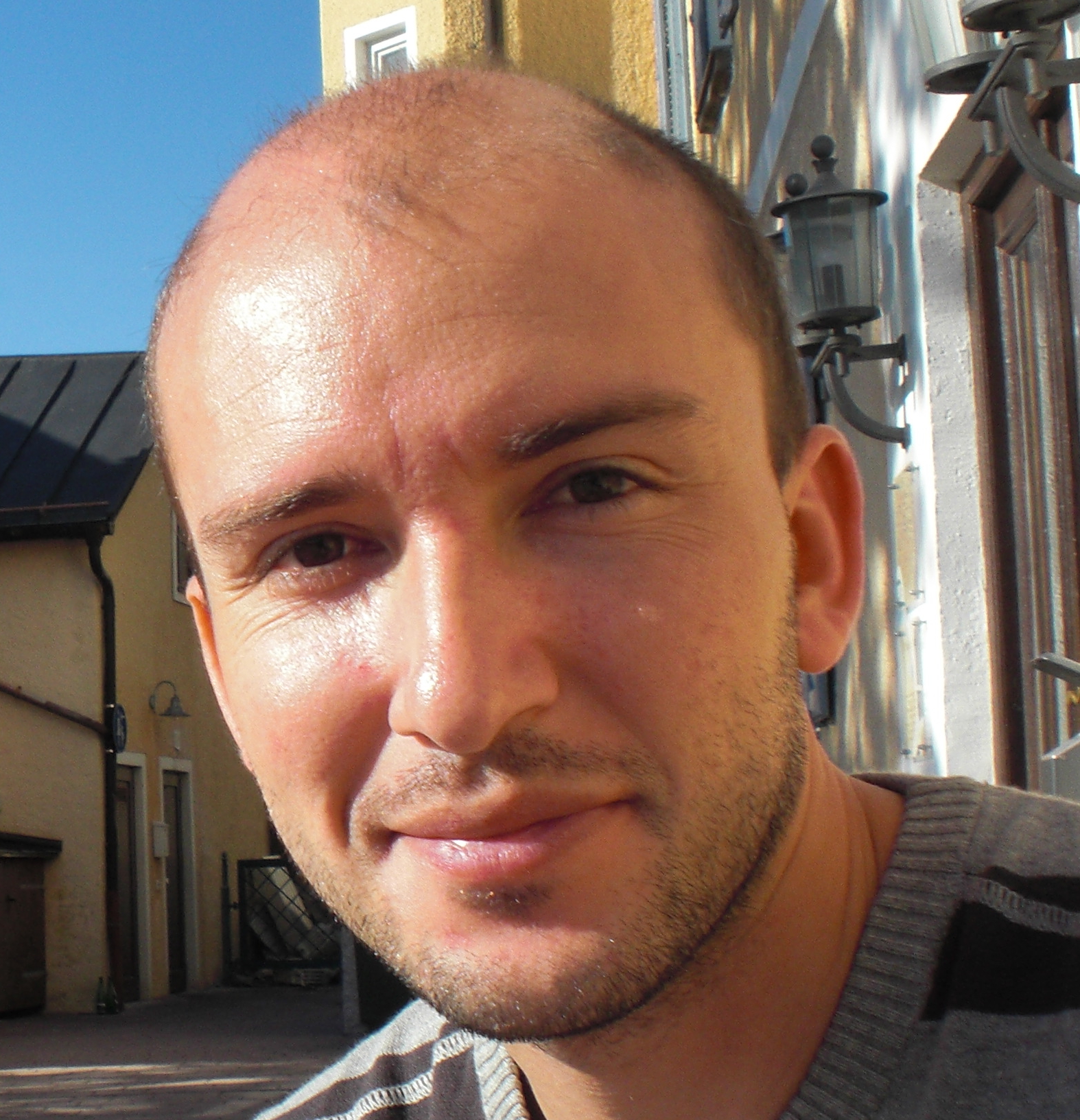
Data compression is an amazing topic. Even in today’s world, with fast networks and almost unlimited storage, data compression is still relevant, especially for mobile devices and countries with poor Internet connections.
For better or worse, GZIP compression is the de-facto lossless compression method for compressing text data in websites. It is not the fastest nor the better, but provides an excellent tradeoff between speed and compression ratio. The way Internet works makes it also difficult to use newer compression methods.
This talk examines how GZIP works internally, explaining the internals of the DEFLATE algorithm, which is a combination of LZ77 and Huffman coding. Different implementations will be compared, such as GNU GZIP, 7-ZIP and zopfli, focusing on why and how some of these implementations perform better than others.
Finally, we will try to go beyond GZIP, preprocessing our data to achieve better results. For example, transposing JSON.
- » Alicante, Spain
- » Website
- » Github
Transcript
Thank you. So hello everyone, my name is Raul. I'm excited to talk to you today. It's my first JSConf 2014 and it is really blowing my mind. So, before getting to topic, let me introduce myself, I come from Spain, where I work as a freelance developer, and I'm also studying masters degree in computer technologies focus on data compression, crip crip and steganography. ‑‑ crypt technology autography. Let's start with data compression, first of all, I'm not an expert on data compression, I am learning about it, and I will like to share with you what I know really. So, for me, data compression is an amazing topic. When I say amazing, it is really amazing, I promise. It can be seen like magic, okay, data compression algorithm take some data and reduce it's size without losing investigation. In some cases like logic compression it may be interesting to lose some information that means once the compressed the result will not be identical. For example in images, sounds, movies, they are more suitable to of use logic compression. On the other hand text is usually compressed using a lossless method like him HTML file ‑‑ it's not magic, we have tools to determine how far we can go in compressing data without loosing information or quantify much information is in a given message. And this is with information theory introduce by Claud etiane nonin 1948 in mathematical theory of communication ‑‑ his famous paper. So, how can we determine how much information is contained in a message? Okay. That answer is Entropy. The Entropy, you can forget the formula for now, the Entropy is the A A amount of information contained in each message. In binary for example, we can concern Entropy the number of bits to represent the message without losing information. The concept of Entropy is quite abstract, and it's not always easy to understand. But, how a message can contain more information than other? Okay, it doesn't make any sense. Imagine for example these two places, Berlin and the Desert of Arizona. We would say it is raining in Berlin it contains less information than if we say it is raining in the Desert of Arizona. That is because it is less common. In Berlin they have 225 days ‑‑ rainy days per year, 62 percent. While in the Desert of Arizona only 17 days, which is six percent. So information theory Entropy is closely related to probabilities, as you can see in the formula. And while this is mostly theoretical our brains are already designed to compress data, we do it all the time. For example, we use multiconstruct to express complex information like feelings with just a couple of characters we can communicate that we're happy, we're sad, we are mocking you. We also have acronyms for common sentences, we no longer say laugh out loud we just say LOL or ASAP for as soon as possible. Savoring time while speaking and saving space when storing it. Even in real life we can share so much information by facial eggs presentations, okay, like the face is telling us if he's safe, happy and having a good time. Of these two examples we could consider a ‑‑ facial eggs presentation as a form of logic compression. Information is being missed here. In the other hand acronyms, if they are unique and well defined, okay, we can decode it uniquely would be a form of lossless compression. But, even before the information theory was developed, data compression was already a key factor for assigning codes to communicate. Take the Morse code for example, to save time and Bandwidth Mors code assigned shorter code to more frequent character ferreters. For example in the English language, the Morse code character ‑‑ the most used character is the letter E, so in Morse it is the shortest code, it is just a dot. So, what about data compression in web sites? Okay, this is the normal flow, okay, the browser sends AEC request to a server to get the file for example index.HTML letting the server know that accept the zip content. The sever then reads the file, compresses on the fly, usually, and sends it to the browser, okay, unless we con fight your the server to ‑‑ content, it does it on the fly. Finally, the browser deCOM presses the file also on the fly, again and gives the reader content. We will see later having to compress and the compress on the fly requires to have a compressional algorithm that ise is fast to compress and fast to decompress in both direction. Let's see how GZIP works internally. GZIP is a file formate, it ise it is a file format based and a compression algorithm, the actual compression takes place in the detailed ‑‑ ‑‑ it was designed by this man, Phil Katz for the PK zip archiving tool and is used for HTTP, PNG and PDF files. Okay. Unfortunately the history of Phil Katz, he was a genius, but he died when he was only 37 due to complications related to chronic alcoholism. So, deplait is a combination of two algorithms, LZ 77 and Hufpmann coding. Let's see how they work. LZ 77 is a losse lossless data compression, we get the original data once compressed. Which is a compression by replacing repeated occurrence of data with references, okay. So, in this example, of we see how the algorithm tries to find repeated occurrences and for example files that space file, space file spice, is spice appears twice. So, replaces the second occurrence with a reference. The first number is the distance, okay, the jump, the number of positions you need to go back to go to the other stream. And the second number is the length, the length of the match. So the decoder can uniquely decode that information. To find matches, the algorithm keeps track of recently read data, of 232 kilobyte, this is called the sliding window. We refer sometimes LZ 77 as a sliding Al gosh rhythm. Once compressed we have three times of data in the compressed stream. We have literals, which would be the characters that have not been replaced by references, we have lengths, the length of the match, and distances, the jump. Okay, and in the second step, the algorithm tries to use ‑‑ bits for the zero, one approach would be to use the necessary bits to represent the symbols. For example here we have the string hello word, we will use ASCII, it uses 8 bits per character, we would need 88 bits to represent that information, that message. As we are only using the characters that appear in the table, they can be represented only with 3 bits, so we can make a transformation and use only 3 bits to represent the message. So, we will of only 33 bits to represent that information. But we can do it better. Instead of fix length codes we can use variable length codes. So, if we ‑‑ if we calculate the frequency, the number of appearances of the characters, we can try to think shorter goals to more frequent symbols like the Morse code does. So, in this example L is used three times. The letter O is just twice, and the others only once. So, we could do this and only use 19 bits instead of 33 to represent the message. Okay, but there is a problem. The compressed stream is ambiguous. These four bits could be, for example, HE, which is correct, A H which is 00 and E which is 01, but it could also be LHO, L 0, H 00 and of O 1. Also the O and many others, so we will need to ‑‑ our next thing is to separate that code using compression ratio. And here is when huffman coding comes, it generates variable length codes, shorter codes to more frequent characters, but they have the prefixed property, the prefixed property says that any of the codes is a prefix of another. So, the output can be decodeble it is not ambiguous. So using huffman coding it can be represented with 32 bits, one bit less than you see in the previous code. And for larger messages gains could be even better. So, Huffman code is used using the compressed output we got from LC 77. One for literals and length and the second for distances. So if we go out now to the GZIP file, not the algorithm, the format file, it compresses an input data in blocks, okay, each block is compressed separately. And there are three modes of compression. In mode one there is no compression at all. It is just useful for data that is already compressed or data that is totally random that cannot be compressed. Mode two uses some already generated Huffman codes using some statistical analysis which fits good enough for most data, and mode three generate those code tables taking into account the data of the block. The mode one is the fastest. And mode three is the slowest, but usually gets better compression ratios. Of of security, you're familiar with ‑‑ you probably have use in the the past to use to split big files into several chunks the size of floppy disks, the non‑compression mode to make these chunks. Okay, so, we just saw how this works you may have noticed this there are different ways to generate the GZIP compliant file, just splitting the file into several blocks with no compression, the no compression mode we get GZIP compliant file, it is really fast but there is no compression at all. And there are a lot of ‑‑ able to create the zip files, each of them use their own optimizations, so the result will be different. Also, there are compression modes, for example the high compression mode spends more time in LC 77 trying to find a longer match, okay. In general 7 zip gets better compression ratio than GNU GZIP, for example Zopfli follows a different approach ‑‑ this is from Google, what it does is spend much more time, when I say much more time, it is much more time, around a hundred times slower to get better compression ratios. Okay, around five percent. This is really useful if we want to compress, for example our JavaScript files or CSS files before deploying, okay, in the deployment process. And then serve those the zip files instead of compressing on the fly. As a general rule, more time, using more time, you get better compression ratios, so you need the find a trade off between doing it off line and up load the compressed files or doing it on the fly. Why GZIP, it is the best compression method? The answer is no. There are many compression algorithms with better compression ratios than GZIP, so why are we still using GZIP? Most of the time in computer science, there are trade offs. GZIP provides good enough compression ratio between 2.5 and 3 for text and it is fast, it is fast to compress data and it is fast to deCOM press it. As we usually can figure out compress responses on the fly, this is something to take into account. In addition, even in the worse case, for example if the data is already compressed, expands the data just a little bit by only five bites by 32‑kilo bytes. We try to compress everything it is important to be sure that damages will be relatively low. Also, the memory needed by ‑‑ the decoder is independent of the size of the data. And finally there are free implementation that avoids ‑‑ it is also difficult to newer compression methods to be widely used. For example the Chromium team tried a few years ago for G.Zip 2 it provides better compression ratio and the results are fast. The problem was that even though the ACTP were correct, and the contents were compressed be B zip 2 the approximateee some of the intermediary proxies didn't understand it. ‑‑ so it was corrupting if data. So, basically, in the short term, at least, we are stuck in the GZIP. What can we do to try to get better compression ratios? So, we can preprocess the data to try to optimize matches. Okay, take a look at this image. This image was generated by a tool of ‑‑ here mall showing what parts are compressed file have been compressed better, those are in blue, and what parts have been expanded. So, we can see the strings of characters in upper case or sometimes the first character of capitalized words expand. Of this is spectral behavior as they are less common and more difficult to find a match to replace with a reference. But preprocess this data to try to optimize the compression and the answer is, yes, sometimes. The goal would be to have a functionality able to transform the data in a way that once is the zip is smaller than the original data is zipped without losing information. And, this depends highly on the type of data we are using. For example there is a technique called transposing JSON, as you know JSON object is made of keycal you pairs where the key portion is repeated for each instance. The basic idea is to group together all the values for each property, so, in the example, the name property will contain all the names and the country property will contain all the countries. Obviously, we will need to change our client‑side JavaScript to be able to read this. But, doing this, we have two main benefits, the first one is that we reduce redene dun Dennis, we are making the files smaller at the expense of spending more time or CPU cycles in the client to interpret that information. But, we are also grouped in similar data together. If you remember when we talked about LC 77 it has a sliding window of 33‑kilo bytes. We make sure that similar data, in this case names or countries will be closed, so it is more likely to find matches between similar data. It is important to note that not always we will better compression ratio with this technique, so we must be careful. For XML and HTML files I have been working on an automatic process in order to order the attributes in a way to maximize LC 77 matches. Here executing executing LC 77, only LC 77, not the detail completely, over the first sample we get an improvement of the file size of 17 percent. As attributes get more ordered, we see the percentage is bigger, so, in general it will follow up to the other attributes, we'll get slightly better results. The process is not as simple as also takes into account the value of the attribute, but, just having a kind of standard to organize your attributes you will get better results. You can see there's a link at the bottom with a small paper on it. So, finally, if you have interest on data compression and GZIP, okay, some recommended material, of compression is a series of videos of data compression, okay. They are done by Colt McAnlis, which makes it easy to understand and funny. So, want to thank him for reviewing this presentation. This is my favorite book on the topic. It is data compression, the complete reference. I am not sure if it's the best idea for a beginner to start with this, but if you're curious to know how a given algorithm ‑‑ the answer is here, the book is quite expensive so ... so if you're not going to read it, don't buy it. And if you like, reading papers I recommend to read this one from Jay cob Zif and abbra ham Lempel where they talk about LC 77. And finally the paper written by David Hutchman on the construction of minimum redundancy codes, it's a very short paper around 12 pages. But the impact on data compression and other fields has been huge. And that's all, thank you, hope you enjoyed (Applause). Edit transcript via pull request.